by Janelle Weaver
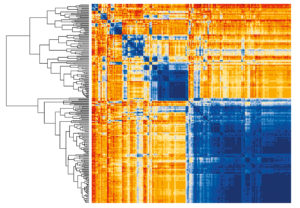
More data is being produced across diverse fields within science, engineering, and medicine than ever before, and our ability to collect, store, and manipulate it grows by the day. With scientists of all stripes reaping the raw materials of the digital age, there is an increasing focus on developing better strategies and techniques for refining this data into knowledge, and that knowledge into action.
Enter data science, where researchers try to sift through and combine this information to understand relevant phenomena, build or augment models, and make predictions.
One powerful technique in data science’s armamentarium is machine learning, a type of artificial intelligence that enables computers to automatically generate insights from data without being explicitly programmed as to which correlations they should attempt to draw.
Advances in computational power, storage, and sharing have enabled machine learning to be more easily and widely applied, but new tools for collecting reams of data from massive, messy, and complex systems—from electron microscopes to smart watches—are what have allowed it to turn entire fields on their heads.
“This is where data science comes in,” says Susan Davidson, Weiss Professor in Computer and Information Science (CIS) at Penn’s School of Engineering and Applied Science. “In contrast to fields where we have well-defined models, like in physics, where we have Newton’s laws and the theory of relativity, the goal of data science is to make predictions where we don’t have good models: a data-first approach using machine learning rather than using simulation.”
Penn Engineering’s formal data science efforts include the establishment of the Warren Center for Network & Data Sciences, which brings together researchers from across Penn with the goal of fostering research and innovation in interconnected social, economic and technological systems. Other research communities, including Penn Research in Machine Learning and the student-run Penn Data Science Group, bridge the gap between schools, as well as between industry and academia. Programmatic opportunities for Penn students include a Data Science minor for undergraduates, and a Master of Science in Engineering in Data Science, which is directed by Davidson and jointly administered by CIS and Electrical and Systems Engineering.
Penn academic programs and researchers on the leading edge of the data science field will soon have a new place to call home: Amy Gutmann Hall. The 116,000-square-foot, six-floor building, located on the northeast corner of 34th and Chestnut Streets near Lauder College House, will centralize resources for researchers and scholars across Penn’s 12 schools and numerous academic centers while making the tools of data analysis more accessible to the entire Penn community.
Faculty from all six departments in Penn Engineering are at the forefront of developing innovative data science solutions, primarily relying on machine learning, to tackle a wide range of challenges. Researchers show how they use data science in their work to answer fundamental questions in topics as diverse as genetics, “information pollution,” medical imaging, nanoscale microscopy, materials design, and the spread of infectious diseases.
Bioengineering: Unraveling the 3D genomic code
Scattered throughout the genomes of healthy people are tens of thousands of repetitive DNA sequences called short tandem repeats (STRs). But the unstable expansion of these repetitions is at the root of dozens of inherited disorders, including Fragile X syndrome, Huntington’s disease, and ALS. Why these STRs are susceptible to this disease-causing expansion, whereas most remain relatively stable, remains a major conundrum.
Complicating this effort is the fact that disease-associated STR tracts exhibit tremendous diversity in sequence, length, and localization in the genome. Moreover, that localization has a three-dimensional element because of how the genome is folded within the nucleus. Mammalian genomes are organized into a hierarchy of structures called topologically associated domains (TADs). Each one spans millions of nucleotides and contains smaller subTADs, which are separated by linker regions called boundaries.
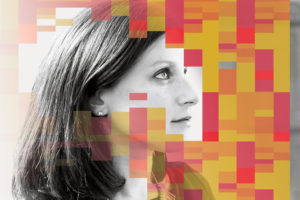
“The genetic code is made up of three billion base pairs. Stretched out end to end, it is 6 feet 5 inches long, and must be subsequently folded into a nucleus that is roughly the size of a head of a pin,” says Jennifer Phillips-Cremins, associate professor and dean’s faculty fellow in Bioengineering. “Genome folding is an exciting problem for engineers to study because it is a problem of big data. We not only need to look for patterns along the axis of three billion base pairs of letters, but also along the axis of how the letters are folded into higher-order structures.”
To address this challenge, Phillips-Cremins and her team recently developed a new mathematical approach called 3DNetMod to accurately detect these chromatin domains in 3D maps of the genome in collaboration with the lab of Dani Bassett, J. Peter Skirkanich Professor in Bioengineering.
“In our group, we use an integrated, interdisciplinary approach relying on cutting-edge computational and molecular technologies to uncover biologically meaningful patterns in large data sets,” Phillips-Cremins says. “Our approach has enabled us to find patterns in data that classic biology training might overlook.”
In a recent study, Phillips-Cremins and her team used 3DNetMod to identify tens of thousands of subTADs in human brain tissue. They found that nearly all disease-associated STRs are located at boundaries demarcating 3D chromatin domains. Additional analyses of cells and brain tissue from patients with Fragile X syndrome revealed severe boundary disruption at a specific disease-associated STR.
“To our knowledge, these findings represent the first report of a possible link between STR instability and the mammalian genome’s 3D folding patterns,” Phillips-Cremins says. “The knowledge gained may shed new light into how genome structure governs function across development and during the onset and progression of disease. Ultimately, this information could be used to create molecular tools to engineer the 3D genome to control repeat instability.”
Read the full story in Penn Today.