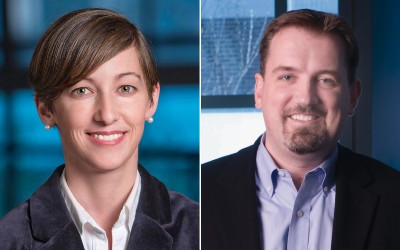
The nature of scientific progress is often summarized by the Isaac Newton quotation, “If I have seen further it is by standing on the shoulders of giants.” Each new study draws on dozens of earlier ones, forming a chain of knowledge stretching back to Newton and the scientific giants his work referenced.
Scientific publishing and referencing has become more formal since Newton’s time, with databases of citations allowing for sophisticated quantitative analyses of that flow of information between researchers.
The Institute for Scientific Information and the Web of Science Group provide a yearly snapshot of this flow, publishing a list of the researchers who are in the top 1 percent of their respective fields when it comes to the number of times their work has been cited.
Danielle Bassett, J. Peter Skirkanich Professor in the departments of Bioengineering and Electrical and Systems Engineering, and Jason Burdick, Robert D. Bent Professor in the department of Bioengineering, are among the 6,389 researchers named to the 2020 list.
Bassett is a pioneer in the field of network neuroscience, which incorporates elements of mathematics, physics, biology and systems engineering to better understand how the overall shape of connections between individual neurons influences cognitive traits. Burdick is an expert in tissue engineering and the design of biomaterials for regenerative medicine; by precisely tailoring the microenvironment within these materials, they can influence stem cell differentiation or trigger the release of therapeutics.
Bassett and Burdick were named to the Web of Science’s 2019 Highly Cited Researchers list as well.
Originally posted in Penn Engineering Today.