by
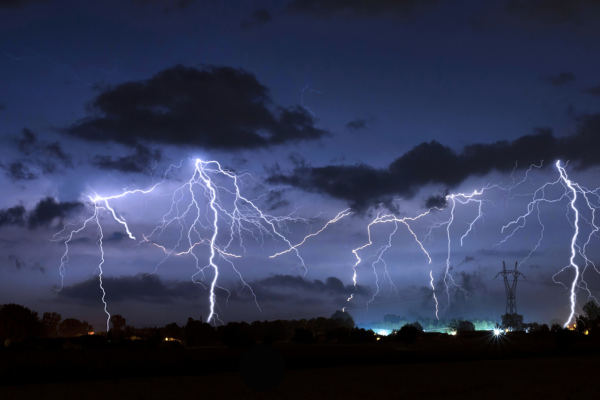
How do we measure chaos and why would we want to? Together, Penn engineers Dani S. Bassett, J. Peter Skirkanich Professor in Bioengineering and in Electrical and Systems Engineering, and postdoctoral researcher Kieran Murphy leverage the power of machine learning to better understand chaotic systems, opening doors for new information analyses in both theoretical modeling and real-world scenarios.
Humans have been trying to understand and predict chaotic systems such as weather patterns, the movement of planets and population ecology for thousands of years. While our models have continued to improve over time, there will always remain a barrier to perfect prediction. That’s because these systems are inherently chaotic. Not in the sense that blue skies and sunshine can turn into thunderstorms and torrential downpours in a second, although that does happen, but in the sense that mathematically, weather patterns and other chaotic systems are governed by physics with nonlinear characteristics.
“This nonlinearity is fundamental to chaotic systems,” says Murphy. “Unlike linear systems, where the information you start with to predict what will happen at timepoints in the future stays consistent over time, information in nonlinear systems can be both lost and generated through time.”
Like a game of telephone where information from the original source gets lost as it travels from person to person while new words and phrases are added to fill in the blanks, outcomes in chaotic systems become harder to predict as time passes. This information decay thwarts our best efforts to accurately forecast the weather more than a few days out.
“You could put millions of probes in the atmosphere to measure wind speed, temperature and precipitation, but you cannot measure every single atom in the system,” says Murphy. “You must have some amount of uncertainty, which will then grow, and grow quickly. So while a prediction for the weather in a few hours might be fairly accurate, that growth in uncertainty over time makes it impossible to predict the weather a month from now.”
In their recent paper published in Physical Review Letters, Murphy and Bassett applied machine learning to classic models of chaos, physicists’ reproductions of chaotic systems that do not contain any external noise or modeling imperfections, to design a near-perfect measurement of chaotic systems to one day improve our understanding of systems including weather patterns.
“These controlled systems are testbeds for our experiments,” says Murphy. “They allow us to compare with theoretical predictions and carefully evaluate our method before moving to real-world systems where things are messy and much less is known. Eventually, our goal is to make ‘information maps’ of real-world systems, indicating where information is created and identifying what pieces of information in a sea of seemingly random data are important.”
Read the full story in Penn Engineering Today.