Pretreatment Determination of Cancer Therapy Efficacy
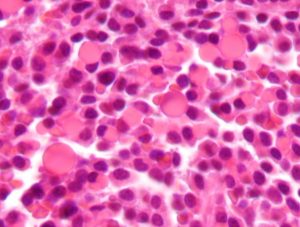
Multiple myeloma is a type of blood cell cancer affecting the blood plasma cells. Although significant advances have improved the treatment of multiple myeloma, the 5-year survival rate remains only 50%. Among the obstacles to increasing survival is that some patients do not respond to drugs for the disease. Similarly, there are only limited ways to predict whether a patient will respond to any given drug.
However, that limitation might be a thing of the past. In a study published recently in Nature Communications, MIT engineers and scientists showed that the mass accumulation rate (MAR) of cancer cells predicted the likelihood of cancerous cells responding to specific drugs. The lead author of the study, Scott R. Manalis of MIT’s Department of Biological Engineering, coauthored the paper with scientists from the Koch Institute for Integrative Cancer Research at MIT, the Dana-Farber Cancer Institute in Boston, and Harvard Medical School.
Dr. Manalis and his colleagues found in previous studies that the MAR, which is the rate at which single cells increase in mass, was predictive of drug sensitivity. The authors used a cleverly designed device called a suspended microchannel resonator to measure the cells’ MAR — itself an impressive feat of engineering given the microscopic size of the myeloma cells. The device was used to analyze multiple myeloma cells obtained from nine patients with the disease. The authors concluded that the MAR could predict the cells’ sensitivity to standard treatment, as well as combination therapies and investigational drugs. If this technology proves effective in larger cohorts, it could significantly increase survival rates for patients with this disease.
Glaucoma Treatment Implant Could Replace Eye Drops
Glaucoma is a common eye disease in which there are abnormal increases in intraocular pressure (IOP), which is a causal predictor for damage to the optic nerve and a precursor to permanent vision loss. Luckily, there are many available treatments for this disease, many of which involve the use of eye drops. However, given the correlation between advanced age and glaucoma, ophthalmologists find that many patients are unable to administer eye drops on their own. Unless they have someone who can administer the drops for them, the patients will lose their vision.
Scientists at the University of California, San Francisco (UCSF), have made a significant advance in solving this problem. The UCSF team, led by Tejal A. Desai, Ph.D., Professor and Chair of the Department of Bioengineering and Therapeutic Sciences, developed a long-term implant for glaucoma patients using polycaprolactone, a type of biodegradable polyester, to eliminate the need for eye drops to treat glaucoma. The authors report in the Journal of Controlled Release that the device could effectively administer a glaucoma drug in rabbits over a six month period. The authors will continue testing, first in larger animals and ultimately, if all goes well, in humans.
Using Deep Learning to Develop Better Microscopes
Artificial neural networks are one type of technology used by scientists to develop machine learning — the process by which computers are designed to learn on their own without being programmed beforehand. In deep learning, a subtype of machine learning, computers process raw data to determine the characteristics they need to know, rather than being “taught.”
The applications of deep learning are potentially limitless. In one application, researchers from the Bioengineering Department at the University of California, Los Angeles (UCLA), are using deep learning to develop more accurate microscopes. Aydogan Ozcan, Ph.D., Chancellor’s Professor and HHMI Professor at UCLA, is lead author of a paper published in Optica describing how he and his colleagues created a deep neural network trained to increase resolution based on visual information. Using images obtained with a regular microscope as their initial data, their network produced significantly higher-quality images that resembled images obtained with higher-magnification lenses. Their findings show that deep learning could improve the quality of low resolution microscopy images, which could significantly enhance point of care applications.
Keeping Bioengineering Ethical
If you’re a frequent reader of this blog, you know we’ve begun producing podcasts. However, a recent podcast produced by Russ Altman, MD, PhD, Professor of Bioengineering, Genetics, Medicine and Biomedical Data Science at Stanford, caught our interest. In the podcast, Dr. Altman interviews Dr. Megan J. Palmer, a Senior Research Scholar at the Center for International Security and Cooperation at Stanford, and they discuss the security challenges faced by scientists involved in biotechnology. Enjoy!
People and Places
Two institutions announced new centers recently for areas related to bioengineering. First, the Texas Medical Center in Houston has opened its Center for Device Innovation — a collaboration between TMC and Johnson & Johnson to facilitate the development of new devices from idea to marketing. In addition, Saint Vincent College near Pittsburgh dedicated a new engineering and biomedical sciences building, the $6 million James F. Will Engineering and Biomedical Science Hall, which will house the college’s biomedical science program.